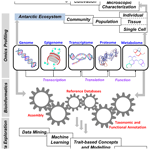
Big data in Antarctic sciences – current status, gaps, and future perspectives
Angelika Graiff
Matthias Braun
Amelie Driemel
Jörg Ebbing
Hans-Peter Grossart
Tilmann Harder
Joseph I. Hoffman
Boris Koch
Florian Leese
Judith Piontek
Mirko Scheinert
Petra Quillfeldt
Jonas Zimmermann
Ulf Karsten
This paper was initiated by a multidisciplinary Topic Workshop in the frame of the Deutsche Forschungsgemeinschaft Priority Program 1158 “Antarctic Research with Comparative Investigations in Arctic Ice Areas”, and hence it represents only the national view without claiming to be complete but is intended to provide awareness and suggestions for the current discussion on so-called big data in many scientific fields.
The importance of the polar regions and their essential role for the Earth system are both undoubtedly recognized. However, dramatic changes in the climate and environment have been observed first in the Arctic and later in Antarctica over the past few decades. While important data have been collected and observation networks have been built in Antarctica and the Southern Ocean, this is a relatively data-scarce region due to the challenges of remote data acquisition, expensive labor, and harsh environmental conditions. There are many approaches crossing multiple scientific disciplines to better understand Antarctic processes; to evaluate ongoing climatic and environmental changes and their manifold ecological, physical, chemical, and geological consequences; and to make (improved) predictions. Together, these approaches generate very large, multivariate data sets, which can be broadly classified as “Antarctic big data”. For these large data sets, there is a pressing need for improved data acquisition, curation, integration, service, and application to support fundamental scientific research. Based on deficiencies in crossing disciplines and to attract further interest in big data in Antarctic sciences, this article will (i) describe and evaluate the current status of big data in various Antarctic-related scientific disciplines, (ii) identify current gaps, (iii) and provide solutions to fill these gaps.
- Article
(3800 KB) - Full-text XML
- BibTeX
- EndNote
The enormous importance of the polar regions has been extensively stressed in the latest Intergovernmental Panel on Climate Change (IPCC) reports (Meredith et al., 2019; IPCC, 2021), where they are clearly recognized as being essential components of the Earth system. The Arctic is warming nearly 4 times faster than the rest of the planet (Rantanen et al., 2022). The melting snow and ice drastically reduces the albedo, leading to darker surfaces and hence increased amounts of solar energy absorbed in these areas. The resulting warming leads to continued loss of sea ice, glaciers, and both ice sheets, with concomitant habitat and biodiversity loss in Arctic sea ice and terrestrial ecosystems (Meredith et al., 2019; Rantanen et al., 2022). Similar trends can be observed particularly around the Antarctic Peninsula (Wille et al., 2022), and in early 2023 Antarctic sea ice extent reached a dramatic all-time low. The regions in which significantly less ice has formed than in the previous year or the long-term average for past years are mainly concentrated in the Bellingshausen Sea and Weddell Sea but also cover broad expanses of Antarctica's eastern coastline (https://www.meereisportal.de, last access: 1 August 2023). Compared to long-term data, an additional area >300 000 km2 was ice-free during the 2023 season. The massive loss of ice and glaciers leads to significant global sea level rise, with strong impacts on coastal regions and cities (Smith et al., 2020; IPCC, 2021). The polar amplification of climate change is a well-known phenomenon already observable and projected by almost all climate models and will intensify over the 21st century, particularly by further substantial warming and increases in precipitation (e.g., Collins et al., 2013; Meredith et al., 2019). Key concerns are manifold and hence there are many approaches spanning multiple scientific disciplines to better understand polar processes; to evaluate ongoing climatic and environmental changes and their ecological, physical, chemical, and geological consequences; and to make (improved) predictions. These approaches, including novel tools for capturing complex ecological scenarios, result in very large, multivariate data sets, which can be broadly classified as “Antarctic big data”.
The term “big data” dates back to the 1990s (reviewed by Diebold, 2012) and usually includes data sets with sizes beyond the ability of commonly used software tools to capture, curate, manage, and process data within a tolerably short time frame (Snijders et al., 2012; Kitchin and Mcardle, 2016; Arribas et al., 2022). Big data encompasses unstructured, semi-structured, and structured data, and requires a set of techniques and technologies with new forms of integration to uncover insights from data sets that are diverse, complex, and of a massive scale. Big data analytics is the process of analyzing big data to extract concealed patterns and information that can yield improved results and new conclusions.
The scientific disciplines addressing research in Antarctica are well developed, and some interdisciplinary approaches already exist, but the latter should be fostered to deepen our knowledge and to obtain a more mechanistic understanding of Antarctic processes, their changes, and their consequences. A representative example is the complex life cycle and ecology of Antarctic krill, which is the most abundant keystone species of the Southern Ocean marine food web and plays an important role in biogeochemical cycles (Cuzin-Roudy et al., 2014; Cavan et al., 2019). Antarctic krill have three critical periods in their early life cycle that strongly affect their survival, with the last one coming during the first winter, when they rely on sea ice biota as food resource and also use sea ice for shelter (e.g., Siegel, 2016). Therefore, environmental conditions such as sea ice quality and quantity and ocean temperature strongly impact the survival of the larvae, meaning that the retreat of winter sea ice and higher temperatures can become dominant drivers of krill population decline. To fully understand and predict the fate of krill populations in the future Southern Ocean, ice-related physical, chemical, and biological expertise is therefore essential. Based on deficiencies in connecting such traditionally separated disciplines and to attract further interest in big data in Antarctic sciences, this article will (i) evaluate the current status of big data in biological, chemical, and geophysical Antarctic science, (ii) identify current gaps, and (iii) provide solutions to fill these gaps.
2.1 Biological view on Antarctic big data – from molecules to ecosystems
Antarctic biodiversity seems to be immense, yet we have only scratched the surface in terms of documenting and understanding many taxonomic groups, such as those in the deep sea or prokaryotic and eukaryotic microorganisms (Gutt et al., 2010; Danis et al., 2020, and references therein). This lack of knowledge is partly related to the difficulty of performing research in harsh environments characterized by extensive sea ice, low temperatures, and long polar nights, which present considerable logistic and infrastructural challenges. Moreover, Antarctica is amongst those areas experiencing the most rapid rates of regional warming (Flexas et al., 2022) and harbors unique ecosystems that are under severe threat from climate change (Pörtner et al., 2023). With regional warming, biotas living in these frozen ecosystems will have to adapt if they are to survive, yet there is currently a very limited understanding of Antarctic biodiversity and even less understanding of the future resilience of these organisms in a changing world. To generate a priori predictions of biodiversity change in Antarctica, it is imperative to understand the true extent of biodiversity, including how organisms interact in food webs, the biological mechanisms by which they have adapted to the cold, their levels of phenotypic plasticity, and how these attributes may impact their abilities to respond to change. Critical to this understanding are a variety of “omics” approaches that exploit the high-throughput sequencing of genetic material.
The so-called omics technologies adopt a holistic view of the genetic repertoire, expression, and analysis of biomolecules that make up a cell, tissue, or organism. They are aimed primarily at the universal detection of genes (genomics), mRNA (transcriptomics), proteins (proteomics), and metabolites (metabolomics) in a specific biological sample across all size classes from bacteria to mammals (e.g., Schneider and Orchard, 2011). Our molecular toolbox has greatly expanded over the past 2 decades as omics techniques have become more advanced and cost-efficient. As a consequence, data wealth has outstripped our capacity to carefully and comprehensively process all molecular information. Analysis of the resulting very large data sets is currently hampered by various bottlenecks, which are discussed below.
All genetic information of any organism is stored in its nucleic acids, DNA and RNA, which can be extracted from individuals of a given species, from entire communities, or even from environmental samples (e.g., from the water column or from a sediment core). These nucleic acid extracts can then be subjected to a variety of high-throughput sequencing approaches, ranging from whole (meta)genome sequencing to (meta)transcriptomic and epigenetic analysis (e.g., Mason et al., 2017). These and related approaches generate huge amounts of nucleotide data, which require quality checking prior to taxonomic or functional analysis. For the latter, numerous automated bioinformatics tools and reference databases have been established, which are essential for processing and evaluating results obtained from massive raw data (Lee et al., 2012; Pappas et al., 2020). Bioinformatics is defined as the application of mathematical and computer science methods to solve problems and address questions in molecular biology that require high-performance computation and analysis (https://www.sib.swiss/what-is-bioinformatics, last access: 1 August 2023).
While the production of massive genetic raw data is relatively easy, their assemblies to genomes or transcriptomes and their interpretation still remain challenging. Genome annotation is the process of identifying and labeling regions of a genome according to their putative functions. Genome annotation, in particular for eukaryotes, remains a major challenge as it is time-consuming and many steps still have to be undertaken manually for quality control (Yandell and Ence, 2012; Salzberg, 2019). Using existing annotations from already described model organisms can be problematic given the diversity of Antarctic organisms and their long histories of evolution in isolation, which in practice tends to result in a situation where only a small proportion of genes can be successfully annotated. Genome annotation is then followed by a comprehensive genomic analysis, which includes the identification, measurement, or comparison of genomic features such as DNA sequences, structural variation, gene expression, or regulatory and functional element annotation at a genomic scale.
Molecular biological databases pervade all areas of bioinformatics. A recent study indicated the existence of over 1700 online molecular biology databases between 1991 and 2016 (Imker, 2018). These include databases on (i) DNA sequences, (ii) predicted protein structures, (iii) phylogenetic trees, (iv) metabolic and regulatory pathways, and (v) gene expression.
Molecular biological data are also important for all ecological questions, since ecology is defined as the complex relationships of organisms to one another and to their physical environment across all scales. Ecological big data systems comprise, for example, in situ and remote sensing data, community-curated data resources, biodiversity databases, citizen science, and long-term networks of ecological monitoring stations. Antarctica is not only underrepresented in these data systems (Li et al., 2020) but also strongly differs in food web structure and dynamics when compared to temperate regions. Nevertheless, rates of ecological data generation, accumulation, and interpretation are continuously growing, with rapid developments in data volumes, methods of data collection, and new analytical and computational approaches. The development and combination of data streams are inspiring because they create new opportunities to study ecological systems at high resolution and on broad temporal and spatial scales, to better understand underlying processes, and to improve ecological forecasting in Antarctica (Dietze, 2017). This evolution in ecological research helps us to fundamentally understand interactions among organisms and with their environments, although the main focus to date has been on temperate to tropical regions.
As ecology is such a diverse and complex scientific discipline, we provide an selected example on animal tracking devices on Antarctic birds, which enable the determination of diurnal and annual movements with increasing accuracy, thereby producing big data. Sensing and tracking technologies are generally becoming cheaper and smaller, producing unprecedented volumes of data for ecological and behavioral studies. In addition to increases in the precision of GPS locators, biologists can now obtain detailed data from bio-logging technologies. Standardized data-analytical pipelines are needed to translate these data into scientific knowledge. A powerful new approach is to assign and analyze behavioral states from accelerometers using machine learning (Fig. 1). Accelerometers measure the inertial acceleration during animal movements, most commonly on three axes. Specific movements are reflected in unique combinations of the three accelerometer axes over time (Fig. 1). From these movements, classes of behavior (e.g., prey captures in penguins) can be identified using support vector machines (SVMs; Carroll et al., 2014; Sutton et al., 2021). A variety of machine learning algorithms have attempted to distinguish among multiple classes of behavior. Two main approaches have been applied to accelerometry data, termed unsupervised and supervised learning methods. In the unsupervised classification approach, accelerometer data are grouped according to similarities in movement patterns using cluster analyses (Sakamoto et al., 2009) or spectral analyses (Ropert-Coudert et al., 2006). However, it has been pointed out that using too many categories of behavior may affect the ability of machine-learning algorithms to accurately classify all behaviors (Ladds et al., 2017). In addition, since different animal species exhibit distinct movement patterns, the optimal machine learning method has to be identified and applied from a set of approaches. Therefore, a “super learning method” has been proposed, which applies sets of candidate machine learning methods to a data set and chooses the optimal model or combination of models (Ladds et al., 2017).
2.2 Chemical view on Antarctic big data
The many different types and large inventories of biological data (Sect. 2.1) are mirrored in analytical and ecological chemistry. Chemistry deals with the properties, composition, quantities, and structure of substances; their transformations; and their thermodynamic budgets. The chemical view on polar science is mainly concerned with measuring and describing very low concentrations of inorganic and organic matter and its transformation along temporal and biological gradients. Moreover, chemistry in ocean sciences is concerned with exact analyses of nutrients, energy compounds and their turnover, elemental stoichiometry of central biological processes, and chemical signalling compounds among and between different kingdoms. Hence, there is a strong linkage between marine chemical data and marine ecology, e.g., when considering nutrients versus primary production.
While by definition big data in natural sciences refers to the collection, processing, and availability of large amounts of data, we also highlight the need for advanced statistics and machine learning tools for the analysis and interpretation of naturally complex chemical samples. Integrating and correlating the wealth of physicochemical data collected by remote observatories and autonomous instruments (e.g., Johnson et al., 2009) with biotic metadata and chemical-analytical data, e.g., data derived by ultra-high-resolution mass spectrometers (Leefmann et al., 2019), is an emerging challenge and opportunity for modern marine chemistry in the polar regions.
Notably, natural mixtures of marine organic molecules exhibit a nearly continuous range of physicochemical properties. Their molecular composition follows a dynamic equilibrium that is shaped by ecosystem characteristics with contributions from biochemical and abiotic (e.g., photo and redox chemistry) reactions. As a consequence, initial signatures of biogenic precursor molecules like lipids, glycans, proteins, and natural products are attenuated beyond recognition, resulting in the most intricate mixtures of organic molecules on Earth. This material represents a valuable inventory of information, transcribing the sum of biotic processes leading to its unique composition.
2.3 Geophysical view on Antarctic big data
Geophysical data are acquired at different levels from ground-based observations, remote controlled or autonomous platforms, and satellite missions (Fig. 2). Here we provide an exemplary perspective on the development and utilization of big data in this scientific discipline without the ambition of completeness.
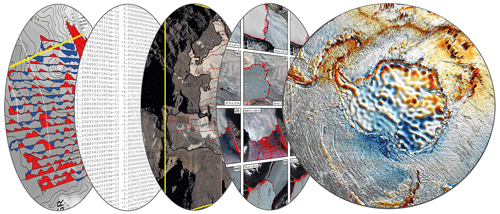
Figure 2Schematic representation of different geophysical data layers and observations from Antarctica in regard to big data.
Since the turn of the century, data from a multitude of new satellite missions have become available. These missions are game changers regarding data availability and access (open and FAIR data access is now developing towards a standard) with exponentially increasing data volumes. Although it is beyond the scope of this paper to review all of these satellite missions, we will highlight a few of them to illustrate their contributions and innovation characteristics and how this is reflected in improvements in data quality and quantity.
In this context, and with respect to environmental monitoring, the European Union/European Space Agency (EU/ESA) Earth observation program Copernicus is most prominent. It provides continuous data acquisition from a fleet of satellites (Sentinels) designed to support a variety of environmental and security purposes and services. A main aspects of the program are its vision for long-term data continuity with constant quality conditions and its expansion by additional new mission concepts. All satellites feature improved radiometric, spatial resolution, and/or spatial coverage. Since the start of data provision in 2013, large databases have been built up according to a coordinated acquisition plan, which is coordinated with missions of other space agencies. Most relevant for polar observations are the synthetic aperture radar (SAR) data from the Sentinel-1 satellites and the multi-spectral imagery of the Sentinel-2 mission. While the Sentinel-2 mission still includes two satellites in the same orbit plane, enabling a 5 d repeat coverage with the same acquisition geometry, one of Sentinel-1 satellites stopped operating in 2022. The satellite Sentinel-1 C is currently being prepared as replacement.
The ESA Earth Explorer Program additionally provides the opportunity to test experimental setups. Within this program, mission concepts like SMOS (Soil Moisture and Ocean Salinity) or CryoSat-2 (Cryosphere) have been developed that are highly relevant for monitoring the Antarctic region via the generation and analysis of data on sea ice thickness. CryoSat-2 altimetry also allows the detection of elevation changes in the continental ice sheets and glaciated areas like the Patagonian ice fields. Its successor, CRISTAL (Copernicus Polar Ice and Snow Topography Altimeter), has been committed for production and launch. Missions like SWARM (constellation of three satellites for mapping Earth's magnetic field) and GOCE (Gravity Field and Steady-State Ocean Circulation Explorer) also provide important information to couple the solid earth and cryosphere, and a follow-up on the gravity mission GOCE is planned by the ESA and NASA for the next decade (MAGIC mission, Mass-change and Geoscience International Constellation), in addition to SCOUT missions that provide shorter-term perspectives for smaller-payload satellite experiments.
Furthermore, national and international satellite missions complement our observational capabilities by deploying specific instruments into orbit. The innovative concept of the German TanDEM-X mission allows for acquiring repeated data to derive global digital elevation models. It provides bistatic interferometric SAR data that are unique regarding their quality, especially in the Antarctic region, where half of the year is spent in darkness and cloud cover is frequent. This allows the determination of glacier-specific elevation changes and sea ice mapping. The Germany–US GRACE and GRACE-FO (Gravity Recovery and Climate Experiment) missions provide unprecedented time series of mass fluxes within the Earth system. For Antarctica, they provide monthly resolution of the mass balance of the continental ice sheets and its interlinkage with global sea level change. In the optical domain, the series of Landsat satellites has been providing data continuity since 1972. Its global archive is open for data access free of charge. In addition, a multitude of small commercial satellites orbit the Earth. Here, the Planet Labs satellites and ICEye have to be mentioned. Although primarily driven by commercial interests, these missions offer scientists access to data on request, exploiting their targeted observation capabilities at high spatial and temporal resolution to supplement the monitoring activities of the large space agencies.
The spaceborne observational capabilities are supported by airborne platforms (planes and drones). Those platforms are more flexible with regard to operation in specific target areas and their payload, which can be adapted to the requirements of specific project tasks. Very often they are equipped with sensors for high-resolution data acquisition, for measurements, or for tests that cannot be performed from space. In that respect and in agreement with the previous sections, we also realize that in geophysics there is a tendency towards considerably increasing data volume sizes.
Ground-based observations are also realized with increased spatial and/or temporal sampling, although access and maintenance still limit continuous operation under polar conditions. These observation systems serve a multitude of applications ranging from inferring meteorological data to GNSS (global navigation satellite system) time series recordings to infer glacial-isostatic adjustment and further geophysical instrumentation for continuous mid- to long-term data acquisitions (e.g., seismometers), various geophysical measurements, and specialized floats and gliders to provide measurements of a variety of ocean parameters (e.g., ARGO).
3.1 Challenges in biology
There is currently a general scarcity of Antarctic genetic data, e.g., from bacterioplankton in the Weddell Sea and other provinces, with even basic taxonomic descriptions of such bacterial communities being missing. The same is true of microorganisms inhabiting Antarctic sediments and all types of ice habitats, while for terrestrial sites the situation is only slightly better. Antarctic deep-sea fauna are also not well addressed in terms of ecology, biodiversity, conservation, taxonomy, and evolutionary biology. The lack of Antarctic microbial data stands in contrast to the better-investigated Arctic regions such as Greenland or Svalbard. In addition, with the third Tara Ocean Expedition in the Arctic (https://fondationtaraocean.org/en/expedition/tara-oceans, last access: 27 July 2033), numerous genetic data sets are now available at least for the plankton, which allow, for example, comparative evaluation of marine microbial biodiversity from other marine provinces (Pesant et al., 2015; Sunagawa et al., 2020; Royo-Llonch et al., 2021; Vernette et al., 2022). One of the scientific highlights was the discovery that one-third of 18S-rDNA sequences could not be assigned to known eukaryotic groups, pointing to a huge unexplored biodiversity, and that most eukaryotic plankton biodiversity belonged to heterotrophic protistan groups, particularly those known to be parasites or symbiotic hosts.
Genome mining describes the exploitation of genomic information for the discovery of biosynthetic pathways of natural metabolites and their possible interactions (Albarano et al., 2020; Chevrette et al., 2021). Genetic and genomic data have been accumulating at unprecedented rates in global sequence databases such as the International Nucleotide Sequence Database Collaboration (INSDC, https://www.insdc.org/, last access: 2 June 2023), but the extraction of well-annotated functional information lags behind. This is mainly because the precise functions of many genes remain unexplored and the time-consuming experimental work to address this problem is not systematically performed. Moreover, often only a subset of the available data can be annotated or used for specific analyses, resulting in the accumulation of large amounts of unused nucleotide data whose potential should be better explored. This is particularly true of samples from less-accessible regions of Antarctica for which reference databases or even sequenced and annotated genomes remain largely incomplete.
As a prerequisite for big data analysis, curated and high-quality databases are essential, but the lack of metadata standards often presents issues (Stow et al., 2018), especially when the inability to taxonomically assign organisms hampers the usability of databases in, for example, biodiversity studies (Bayraktarov et al., 2019; Loeffler et al., 2021). Many data archives also suffer from the fact that submitters do not always adhere even to minimal sequence metadata standards. Often elementary information is missing, such as the GIS (geographic information system) coordinates of sample locations. Additionally, nomenclatural databases should be interlinked to sequence databases as an important prerequisite for the sound use of big data. In open-access data centers such as PANGAEA, there is the problem that authors submitting data sometimes use different names for the same entity, and as a result the data managers often cannot sort them out.
3.2 Challenges in chemistry
The concept of deciphering natural chemical complexity (chemodiversity) is rather new to chemistry, a field of science that traditionally attempts to predict and control reactions, kinetics, and products rather than to characterize complex molecular mixtures containing thousands of different molecules. Especially in the life sciences, this is rapidly changing, with systems chemistry (analogous to systems biology) and environmental metabolomics emerging as powerful strategies to capture and interpret biological processes and their chemical fingerprints at various levels. As ecosystems are transformed on different timescales to complex organic matter and geopolymers, in marine biogeochemistry this approach is emerging as the strategy to understand contemporary and historical processes.
Novel instrumental analytical technologies enable the partial description of this chemical diversity (Steen et al., 2020). The main challenge is the integration, processing, and validation of the chemical data produced by such instruments in order to distinguish and map the plethora of molecular formulae and (if possible) to derive structural molecular information (Leefmann et al., 2019). Mathematics and statistics offer important opportunities to understand these data and to provide context in terms of significant chemical and/or biological information. Chemometrics are valuable to mine correlated information (e.g., biotic and abiotic environmental parameters, species abundance, genetic repertoires, and gene expression) and to decipher multidimensional analytical spectra and data. For the investigation of complex natural mixtures, a systems approach using a variety of chemical and bioanalytical approaches is the key. Such systems chemistry strategies are currently emerging and involve state-of-the-art selective separation combined with high-resolution spectral and/or spectrometric technologies. They are complemented by extensive chemical big-data mining and most recently supported by machine learning.
3.3 Challenges in geophysics
The resulting amounts of data are steadily growing, which allows the analysis of time series that cover decadal time spans. It fosters the development and application of new methods in the analysis of big data. Often, a combination of different sensors at the same platform and/or at different levels from ground-based to airborne and spaceborne instruments enables improved insights but also increases the volume of data to be handled. Today, not only in Earth sciences, we are facing such huge data volumes that we urgently need to improve already existing processing and to develop new, automated processing routines by applying fast and efficient algorithms. Artificial intelligence (AI) provides novel methods for data combination to exploit the information content to an optimum and to improve the quality and significance of research outcomes (Baumhoer et al., 2019; Davari et al., 2021; Loebl et al., 2022). However, those methods require a large amount of training and validation data that might not be available in the environmental sciences, especially in Antarctica. Hence, these data may need to be generated specifically. In addition, such data volumes require respective processing facilities (e.g., high-performance computing, HPC) together with adequate and fast storage. While most research institutions have access to such HPC processing facilities, data sets are often stored or at least downloaded various times at multiple locations to facilitate processing and increase network traffic. However, the need to re-access data to restore time series severely limits the reprocessing of archives applying new software versions or novel algorithms when new product lines are inferred using complete data time series.
A variety of examples for large-scale automated processing and data dissemination for the Antarctic region are already in place, e.g., the Alfred Wegener Institute/University of Bremen sea ice portal (https://www.meereisportal.de/, last access: 1 August 2023), the glacier portal of the Friedrich-Alexander-University Erlangen-Nürnberg (Friedl et al., 2021), or the Technical University Dresden geodetic data portal (Groh and Horwath, 2021). Those portals have evolved out of single or consecutive project activities that have been heavily used at both the national and international level. However, maintaining and updating such portals is normally beyond the scope and capabilities of small university research groups, which tend to have limited core resources. In addition, such activities are in general not supported by many funding schemes. As a consequence, data product lines or prototype services are often discontinued or only reworked when new project funds allow it. There is also no mechanism to transfer such established product lines to a continuous operation, e.g., at a national institution or a Copernicus service. Commercial operators face the same problem and, in addition, have larger operation costs since HPC access has to be purchased. This is a major setback in view of the need to continue time series records for the evaluation of changes in the Antarctic region, specifically in view of climate change scenarios. Furthermore, research policy asks for more and better science communication. Access to science products with respective quality assurance accompanied by some guidance on usage for either direct access by the general public science journalists or for institutions of secondary and higher education would be a strong building block for an informed public and will also help to raise awareness and interest for the next generation of scientists.
In addition, the modeling community faces similar difficulties to the remote sensing groups. The spatial and temporal resolutions of models and their extent are steadily being improved, which is increasing demand for processing and archiving capabilities.
4.1 Perspectives in biology
Based on the current status of Antarctic research and the above identified knowledge gaps, we have formulated the following recommendations. Big data analyses in Antarctic research crucially rely upon high-quality databases, which should be peer reviewed and curated by experts in their respective fields. High-quality, contiguous reference genomes are also needed, and ideally these should be annotated to a high and eventually even internationally universal standard. Thus, an initiative to produce high-quality reference genomes for a representative selection of Antarctic organisms would be desirable.
We also strongly recommend the use of standardized vocabulary such as Environmental Ontology (EnvO) (https://sites.google.com/site/environmentontology/, last access: 2 June 2023) for creating (meta)data sets. This would improve data quality, as ontological software understands the different terms and recognize synonyms, as well as facilitate the machine reading of entries, which is important for data mining and machine learning. Consequently, international standards for the preparation and submission of molecular data, metadata, and GIS-referenced data into a single database are essential. For this, the FAIR data standards and FAIR principles (Findable, Accessible, Interoperable and Reusable) for digital assets have been established (https://www.go-fair.org/fair-principles/, last access: 2 June 2023). These principles emphasize machine action ability, i.e., the capacity of computational systems to find, access, inter-operate, and reuse data with no or minimal human intervention, because scientists rely more and more on computational pipelines to deal with big data due to rapid increases in volume, complexity, and creation speed (Tanhua et al., 2019). The FAIR principles should of course be applied equally to all Antarctic scientific disciplines.
A further important aspect is the need to harmonize molecular biological workflows and bioinformatics pipelines to provide optimized tools for big data analysis to the scientific community. In addition, improved communication between various existing databases would again be desirable for harmonization but also for interlinkage and comparison. A good example is GBIF (Global Biodiversity Information Facility, https://www.gbif.org, last access: 2 June 2023) as an international network and data infrastructure, which could still be improved despite already having numerous links to other databases. Such approaches would be a great step forward for big data analyses and synthesis of the Antarctic region.
Since raw molecular data are often processed differently by scientists, it is also important to understand the conceptual choices influencing the production and classification of the results. For example, the outcome of raw data analysis can be impacted by filtering steps and software version updates. Hence, we recommend that omics papers should always provide such information, ideally accompanied by the code used to implement the original analyses. This would greatly improve reproducibility and comparability to other studies.
Greater emphasis should also be placed on the generation and maintenance of long time series of genomic data sets, which are clearly essential for assessing the impacts of current and future environmental change. Without baseline information, e.g., on microbial communities, it will be challenging if not impossible to monitor and analyze temporal changes.
Improving our fundamental understanding of Antarctic biodiversity and the molecular mechanisms underlying environmental and evolutionary acclimation and adaptation is essential for predicting future changes in the structure and function of marine, freshwater, and terrestrial communities in Antarctica in relation to ongoing climate change. In particular, a systems biology approach is needed to understand the manifold complex genomic, transcriptomic, proteomic, and metabolomic interactions on the species, population, and community level, which is necessary for achieving a holistic understanding of feedbacks between biota and their (changing) environment or even how biota change their environments. This should also include new model organisms capable of representing past and current biotic and abiotic effects on various ecosystem services such as biodiversity, primary production, and biogeochemical cycling, as well as predicting such functions in the future. The latest Tara Ocean Expedition in the Arctic could serve as a template, and big data analysis would be essential to better understand and interpret the massive amount of raw data. Such studies might also provide an opportunity not only to discover organisms that are new to science but also to characterize new genes, metabolic pathways, and biotic interactions, all of which contribute to better understanding the contemporary and future biology of the Antarctic region (Fig. 3).
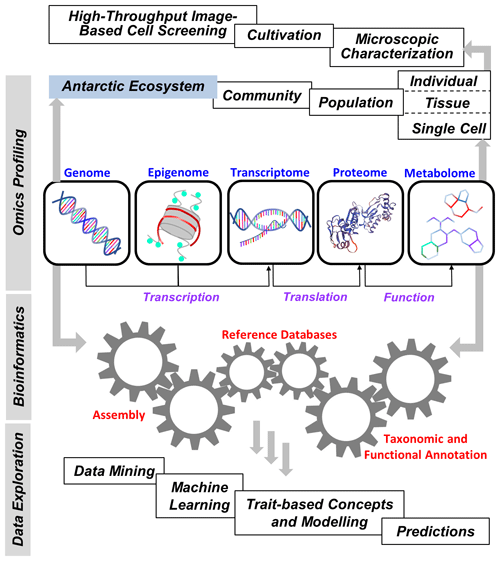
Figure 3Elements of “omics” in polar big data science. Flow of genetic techniques, omics approaches, bioinformatics, and final data exploration to provide a better understanding of the current and future biological diversity and processes of the Antarctic ecosystem.
Based on currently available ecological big data, we recommend focusing on integrating ecosystem processes, structures, and functions and their responses and adaptations to global changes at the regional and global scale. The main objectives are to quantitatively assess and scientifically predict the responses of ecosystem components and key processes to climate and environmental changes. In principle, the research methods of integrative ecology include meta-analysis, data mining, and data–model synthesis. However, big data can be challenging to work with due to large data volumes, heterogeneity in data quality and uncertainty, and the need for metadata as described earlier (Yang and Huang, 2013; Ladeau et al., 2017; Chang and Grady, 2019). None of these challenges are entirely new to ecologists, but the massive increase in data in all four dimensions challenges traditional approaches to data management and analysis.
To analyze large and detailed data sets, machine learning techniques (reviewed in Valletta et al., 2017) are ideally suited to the task of extracting biological insights from complex behavioral and observational data, as well as from large data sets such as digital images and video recordings. Machine learning techniques including support vector machines (SVMs), classification and regression trees (CART), and artificial neural networks (ANNs) provide computationally powerful methods of data classification. Thus, machine learning is expected to play a central role in extracting scientific knowledge from big data in ecology.
Technological solutions to deal with big data include the development of open code- and data-sharing platforms, flexible statistical models that can handle heterogeneous data and sources of uncertainty and cloud-computing delivery of high-velocity computing. Educational solutions include providing training to both established and early career scientists and strengthening collaborations between biologists and data scientists. The broader goal is to maximize the power, scalability, and timeliness of biological insights and ecological forecasting. Hence, Antarctic big data should be considered an almost unexplored treasure containing massive amounts of hidden information waiting to be extracted and interpreted by smart technologies and by well-educated, interdisciplinary scientists.
In recent years, some national and international programs have begun to address the problems described in this paper. On the national level there is, for example, the NFDI4Biodiversity consortium (https://www.nfdi4biodiversity.org, last access: 12 July 2023), which is hosted under the umbrella of the National Research Data Infrastructure (NFDI). NFDI4Biodiversity is dedicated to the mobilization of biodiversity and environmental data for collective use according the FAIR principles. In NFDI, valuable data resources for the German science system are systematically made accessible, federated, and usable in a sustainable way. The main goal of this consortium is to provide scientific and technical competencies to users from research and practice with a service portfolio for biodiversity and environmental data. On the European level, various programs have also been established, such as Blue-Cloud (https://blue-cloud.org, last access: 12 July 2023). The mission of Blue-Cloud is to provide a virtual environment with open and seamless services for the storage, management, analysis, and reuse of research data across borders and disciplines. On the international level, the activities of the Joint Genome Institute (JGI), U.S. Department of Energy (https://jgi.doe.gov, last access: 2 June 2023), developed a vision for integrative and collaborative genome science. Their mission is to provide the global research community with access to the most advanced integrative genome science capabilities including state-of-the-art technologies and user-friendly data portals. JGI will develop and employ advanced and accessible computational approaches to enable the integrative analysis and interpretation of multiple orthogonal data types including transcriptomic, epigenomic, and metabolomics results in conjunction with genomic information.
4.2 Perspectives in chemistry
The analytical strategies outlined above present powerful opportunities to investigate the structure, origin, transformation, ecological function, and distribution of organic compounds under changing conditions in the polar oceans. The Southern Ocean Observing System (SOOS, https://www.scar.org/soos/, last access: 1 August 2023; Meredith et al., 2013) and the ARGO float program (https://www.ocean-ops.org/board?t=argo, last access: 1 August 2023; Roemmich et al.; 2009, Boening et al., 2008) are good examples of initiatives that facilitate the integration of multiple resources to enhance the analysis and interpretation of physicochemical data. The availability of high-quality, long-term observational data and the establishment of open-access data centers such as PANGAEA (https://www.pangaea.de/, last access: 1 August 2023; Dittert et al., 2002; Diepenbroek et al., 2002) or the World Ocean Database (WOA; https://www.ncei.noaa.gov/products/world-ocean-database, last access: 1 August 2023) enable chemical ocean data to be shared in larger, increasingly global contexts. Open access to these data repositories is critical to fostering scientific collaboration and progress. Moreover, the broad accessibility of data stimulates and enables research independent of traditional funding models (Levine et al., 2020). As a result of these efforts over the past 2 decades, automation in ocean sensor techniques and data acquisition in the laboratory in combination with elegant data archives has dramatically increased the size of data sets available to address research questions relating to the polar oceans and beyond.
4.3 Perspectives in geophysics
We recommend establishing a central European archive for satellite data including processing facilities with access via a data hub for national institutions. Current solutions do not hold all of the required data from Antarctica (and not even from the Sentinel satellite series), are not accessible to all polar researchers, or impose costs when considering long-term activities. Especially university groups, which cannot base their Antarctic research on core funding, face limits regarding regular processing and service. Here, new funding lines and/or operation modes to access respective data are required, together with adequate processing infrastructure. While requirements with regard to the long-term archiving of the results of research have found their way into proposals, the related facilities and associated costs are often not in place or are not sufficiently covered at most institutions to handle the enormous amount of data. Here, we see a strong need for a coordinated effort under the NFDI4Earth initiative to consider and include such large data sets at the HPC level.
Quality assurance and proper metadata documentation for subsequent usage are further challenges that arise from automated processing. The assimilation of automated data into geophysical models requires information on the product quality and reliability. Continuous data flow, as outlined above, would be desirable as an additional incentive for efforts to accordingly adapt models. To date, not all Earth observation products can be readily assimilated into respective models. Here, closer interactions among the respective research communities are required, as are intelligent assimilation strategies.
Antarctica harbors some of the least well-known biodiversity on Earth and is currently facing dramatic changes in the climate and environment. Although important (big) data have been collected and observation networks have been built in Antarctica and the Southern Ocean, this region is still relatively data scarce due to the challenges of remote data acquisition, expensive labor, and harsh environmental conditions. For example, winter sampling campaigns are missing so far. There are many approaches crossing multiple scientific disciplines to better understand Antarctic processes; to evaluate ongoing climatic and environmental changes and their manifold ecological, physical, chemical, and geological consequences; and to make (improved) predictions. Together, these approaches generate very large, multivariate data sets, which can be broadly classified as “Antarctic big data”. For these large data sets, there is a pressing need for improved data acquisition, curation, integration, service, and application to support fundamental scientific research.
Although NFDI4Biodiversity and other initiatives provide intensive training programs for students, we consider the interface between biology and bioinformatics to still be underdeveloped. Hence, this needs to be more strongly integrated into the curricula of German universities. The majority of research (at least in the university setting) is performed by doctoral students, many of whom lack even a basic grounding in bioinformatics at the start of their PhDs. More training courses, such as in NFDI4Biodiversity, and dedicated support linked to bioinformatics concepts and infrastructures would help these young scientists to acquire the necessary skills to analyze their data more quickly and effectively. Therefore, specific masters courses across disciplines are urgently needed. Better education and training of the future generation of polar scientists is essential if we as a community are to seamlessly embed big data across the traditional domains of physical oceanography, marine biology, chemistry, geophysics, and geology. A recent dedicated initiative in this direction is the new “Helmholtz Graduate School for Marine Data Science” (MarDATA; https://www.mardata.de/, last access: 1 August 2023; Verwega et al., 2021).
AI techniques promise improved performance but should not reduce the capabilities of the models to simulate the underlying processes and mechanisms. We see a strong demand for cross-disciplinary research and interaction between computer scientists, data scientists, and environmental scientists to address these requirements. Both technical skills and a fundamental understanding of the environmental problems and processes within the Earth system are required. Hence, we suggest in line with the biological and chemical sections to that there should be more interdisciplinary education efforts, particularly at the masters and PhD levels (e.g., International Doctoral Program MOCCA within the Elitenetzwerk Bayern).
With such an educational effort Antarctica will be more effectively integrated into biological, chemical, and geophysical big data systems, thereby addressing significant knowledge gaps between currently observed changes and the underlying mechanisms.
No data sets were used in this article.
AG and UK developed the structure and concept based on contributions from all other authors. All authors edited and improved the first draft, contributed to the revision, and approved the final submission.
The contact author has declared that none of the authors has any competing interests.
Publisher's note: Copernicus Publications remains neutral with regard to jurisdictional claims in published maps and institutional affiliations.
The paper was supported by the DFG Priority Program 1158 “Antarctic Research with Comparative Investigations in Arctic Ice Areas”.
This research has been supported by the Deutsche Forschungsgemeinschaft (DFG Priority Program 1158 “Antarctic Research with Comparative Investigations in Arctic Ice Areas”).
This paper was edited by Bernhard Diekmann and reviewed by two anonymous referees.
Albarano, L., Esposito, R., Ruocco, N., and Costantini, M.: Genome Mining as New Challenge in Natural Products Discovery, Mar. Drugs, 18, 199, https://doi.org/10.3390/md18040199, 2020.
Arribas, P., Andújar, C., Bohmann, K., deWaard, J. R., Economo, E. P., Elbrecht, V., Geisen, S., Goberna, M., Krehenwinkel, H., Novotny, V., Zinger, L., Creedy, T. J., Meramveliotakis, E., Noguerales, V., Overcast, I., Morlon, H., Papadopoulou, A., Vogler, A. P., and Emerson, B. C.: Toward global integration of biodiversity big data: a harmonized metabarcode data generation module for terrestrial arthropods, GigaScience, 11, giac065, https://doi.org/10.1093/gigascience/giac065, 2022.
Baumhoer, C., Andreas, D., Kneisel, C., and Kuenzer, C.: Automated Extraction of Antarctic Glacier and Ice Shelf Fronts from Sentinel-1 Imagery Using Deep Learning, Remote Sens., 11, 1–22 https://doi.org/10.3390/rs11212529, 2019.
Bayraktarov, E., Ehmke, G., O'Connor, J., Burns, E. L., Nguyen, H. A., McRae, L., Possingham, H. P., and Lindenmayer, D. B.: Do Big Unstructured Biodiversity Data Mean More Knowledge? Front. Ecol. Evol., 6, 239, https://doi.org/10.3389/fevo.2018.00239, 2019.
Boening, C., Dispert, A., Visbeck, M., Rintoul, S. R., and Schwarzkopf, F. U.: The response of the Antarctic Circumpolar Current to recent climate change, Nat. Geosci., 1, 864–869, https://doi.org/10.1038/ngeo362, 2008.
Carroll, G., Slip, D. J., Jonsen, I., and Harcourt, R. G.: Supervised accelerometry analysis can identify prey capture by penguins at sea, J. Exp. Biol., 217, 4295–302, https://doi.org/10.1242/jeb.113076, 2014.
Cavan, E. L., Belcher, A., Atkinson, A., Hill, S. L., Kawaguchi, S., McCormack, S., Meyer, B., Nicol, S., Ratnarajah, L., Schmidt, K., Steinberg, D. K., Tarling, G. A., and Boyd, P. W.: The importance of Antarctic krill in biogeochemical cycles, Nat. Commun., 10, 4742, https://doi.org/10.1038/s41467-019-12668-7, 2019.
Chang, W. and Grady, N.: NIST Big Data Interoperability Framework: Volume 1, Definitions, Special Publication (NIST SP), National Institute of Standards and Technology, US Department of Commerce, https://doi.org/10.6028/NIST.SP.1500-1r2, 2019.
Chevrette, M. G., Gavrilidou, A., Mantri, S., Selem-Mojica, N., Ziemert, N., and Barona-Gòmez, F.: The confluence of big data and evolutionary genome mining for the discovery of natural products, Nat. Prod. Rep., 38, 2024–2040, https://doi.org/10.1039/D1NP00013F, 2021.
Collins, M., Knutti, R., Arblaster, J., Dufresne, J.-L., Fichefet, T., Friedlingstein, P., Gao, X., Gutowski, W. J., Johns, T., Krinner, G., Shongwe, M., Tebaldi, C., Weaver A. J., and Wehner, M.: Long-term Climate Change: Projections, Commitments and Irreversibility, in: Climate Change 2013, the Physical Science Basis, Contribution of Working Group I to the Fifth Assessment Report of the Intergovernmental Panel on Climate Change, edited by: Stocker, T. F., Qin, D., Plattner, G.-K., Tignor, M., Allen, S. K., Boschung, J., Nauels, A., Xia, Y., Bex, V., and Midgley, P. M., Cambridge University Press, Cambridge, United Kingdom and New York, NY, USA, 2013.
Cuzin-Roudy, J., Irisson, J. O., Penot, F., Kawaguchi, S., and Vallet, C.: Southern Ocean Euphausiids, in: Biogeographic Atlas of the Southern Ocean, edited by: De Broyer, C., Koubbi, P., Griffiths, H. J., Raymond, B., Udekem d'Acoz, C., Van de Putte, A. P., Danis, B., David, B., Grant, S., Gutt, J., Held, C., Hosie, G., Huettmann, F., Post, A., and Ropert-Coudert, Y., Scientific Committee on Antarctic Research, Cambridge, 309–320, ISBN 978-0-948277-28-3, 2014.
Danis, B., Van de Putte, A., Convey, P., Griffiths, H., Linse, K., and Murray, A.E.: Editorial: Antarctic Biology – Scale Matters, Front. Ecol. Evol. 8, 91, https://doi.org/10.3389/fevo.2020.00091, 2020.
Davari, A., Islam, S., Seehaus, T., Hartmann, A., Braun, M., Maier, A., and Christlein, V.: On Mathews Correlation Coefficient and Improved Distance Map Loss for Automatic Glacier Calving Front Segmentation in SAR Imagery, IEEE T. Geosci. Remote, 1–12, https://doi.org/10.1109/TGRS.2021.3115883, 2021.
Diebold, F. X.: A Personal Perspective on the Origin(s) and Development of `Big Data': The Phenomenon, the Term, and the Discipline, Second Version, PIER Working Paper No. 13-003, https://doi.org/10.2139/ssrn.2202843, 26 November 2012.
Dietze, M. C.: Ecological Forecasting, Princeton University Press, 288 pp., ISBN 9780691160573, 2017.
Dittert, N., Corrin, L., Diepenbroek, M., Grobe, H., Heinze, C., and Ragueneau, O.: Management of (pale-) oceanographic data sets using the PANGAEA information system: the SINOPS example, Comput. Geosci., 28, 789–798, https://doi.org/10.1016/S0098-3004(01)00112-1, 2002.
Flexas, M. M., Thompson, A. F., Schodlok, M. P., Zhang, H., and Speer, K.: Antarctic Peninsula warming triggers enhanced basal melt rates throughout West Antarctica, Sci. Adv. 8, eabj9134, https://doi.org/10.1126/sciadv.abj9134, 2022.
Friedl, P., Seehaus, T., and Braun, M.: Global time series and temporal mosaics of glacier surface velocities derived from Sentinel-1 data, Earth Syst. Sci. Data, 13, 4653–4675, https://doi.org/10.5194/essd-13-4653-2021, 2021.
Groh, A. and Horwath, M.: Antarctic Ice Mass Change Products from GRACE/GRACE-FO Using Tailored Sensitivity Kernels, Remote Sens., 13, 1736, https://doi.org/10.3390/rs13091736, 2021.
Gutt, J., Hosie, G., and Stoddart, M.: Marine Life in the Antarctic, in: Life in the world's oceans: diversity, distribution, and abundance, edited by: McIntyre, A. D., Wiley Blackwell, Oxford, 203–220, 2010.
Imker, H. J.: 25 Years of Molecular Biology Databases: A Study of Proliferation, Impact, and Maintenance, Front. Res. Metr. Anal., 3, 18, https://doi.org/10.3389/frma.2018.00018, 2018.
IPCC: Climate Change 2021, The Physical Science Basis, Contribution of Working Group I to the Sixth Assessment Report of the Intergovernmental Panel on Climate Change, edited by: Masson-Delmotte, V., Zhai, P., Pirani, A., Connors, S. L., Péan, C., Berger, S., Caud, N., Chen, Y., Goldfarb, L., Gomis, M. I., Huang, M., Leitzell, K., Lonnoy, E., Matthews, J. B. R., Maycock, T. K., Waterfield, T., Yelekçi, O., Yu, R., and Zhou, B., Cambridge University Press, Cambridge, United Kingdom, 2391 pp., https://doi.org/10.1017/9781009157896, 2021.
Johnson, K. S., Berelson, W. M., Boss, E. S., Chase, Z., Claustre, H., Emerson, S. R., Gruber, N., Kortzinger, A., Perry, M. J., and Riser, S. C.: Observing Biogeochemical Cycles at Global Scales with Profiling Floats and Gliders Prospects for a Global Array, Oceanogr., 22, 216–225, https://doi.org/10.5670/oceanog.2009.81, 2009.
Kitchin, R. and McArdle, G.: What makes Big Data, Big Data? Exploring the ontological characteristics of 26 datasets, Big Data Soc., 3, 1–10, https://doi.org/10.1177/2053951716631130, 2016.
Ladds, M. A., Thompson, A. P., Kadar, J.-P., Slip, D. J., Hocking, D. P., and Harcourt, R. G.: Super machine learning: improving accuracy and reducing variance of behaviour classification from accelerometry, Anim. Biotelemetry, 5, 8, https://doi.org/10.1186/s40317-017-0123-1, 2017.
LaDeau, S. L., Han, B. A., Rosi-Marshall, E. J., and Weathers, K. C.: The next decade of big data in ecosystem science, Ecosyst., 20, 274–283, https://doi.org/10.1007/s10021-016-0075-y, 2017.
Lee, H. C., Lai, K., Lorenc, M. T., Imelfort, M., Duran, C., and Edwards, D.: Bioinformatics tools and databases for analysis of next-generation sequence data, Brief. Funct. Genom., 11, 12–24, https://doi.org/10.1093/bfgp/elr037, 2012.
Leefmann, T., Frickenhaus, S., and Koch, B.P.: UltraMassExplorer: a browser-based application for the evaluation of high-resolution mass spectrometric data, Rapid Commun. Mass Spectrom., 33, 193–202, https://doi.org/10.1002/rcm.8315, 2019.
Levine, R. M., Fogaren, K. E., Rudzin, J. E., Russoniello, C. J., Soule, D. C., and Whitaker, J. M.: Open Data, Collaborative Working Platforms, and Interdisciplinary Collaboration: Building an Early Career Scientist Community of Practice to Leverage Ocean Observatories Initiative Data to Address Critical Questions in Marine Science, Front. Mar. Sci., 7, https://doi.org/10.3389/fmars.2020.593512, 2020.
Li, X., Che, T., Li, X., Wang, L., Duan, A., Shangguan, D., Pan, X., Fang, M., and Bao, Q.: CASEarth Poles: Big Data for the Three Poles, Bull. Am. Meteorol. Soc., 101, 1475–1491, https://doi.org/10.1175/BAMS-D-19-0280.1, 2020.
Loebl, E., Scheinert, M., Horwath, M., Heidler, K., Christmann, J., Phan, L. D., Humbert, A., and Zhu, X. X: Extracting Glacier Calving Fronts by Deep Learning: The Benefit of Multispectral, Topographic, and Textural Input Features, IEEE T. Geosci. Remote, 60, 4306112, https://doi.org/10.1109/TGRS.2022.3208454, 2022.
Loeffler, F., Wesp, V., König-Ries, B., and Klan, F.: Dataset search in biodiversity research: Do metadata in data repositories reflect scholarly information needs?, PLoS ONE, 16, e0246099, https://doi.org/10.1371/journal.pone.0246099, 2021.
Mason, C. E., Afshinnekoo, E., Tighe, S., Wu, S., and Levy, S.: International Standards for Genomes, Transcriptomes, and Metagenomes, J. Biomol. Tech., 28, 8–18, https://doi.org/10.7171/jbt.17-2801-006, 2017.
Meredith, M., Sommerkorn, M., Cassotta, S., Derksen, C., Ekaykin, A., Hollowed, A., Kofinas, G., Mackintosh, A., Melbourne-Thomas, J., Muelbert, M. M. C., Ottersen, G., Pritchard, H., and Schuur, E. A. G.: Polar Regions, in: IPCC Special Report on the Ocean and Cryosphere in a Changing Climate, edited by: Pörtner, H.-O., Roberts, D.C., Masson-Delmotte, V., Zhai, P., Tignor, M., Poloczanska, E., Mintenbeck, K., Alegría, A., Nicolai, M., Okem, A., Petzold, J., Rama, B., and Weyer, N. M., Cambridge University Press, Cambridge, United Kingdom, 203–320, https://doi.org/10.1017/9781009157964.005, 2019.
Meredith, M. P., Schofield, O., Newman, L., Urban, E., and Sparrow, M.: The vision for a Southern Ocean Observing System, Curr. Opin. Environ. Sustain., 5, 306–313, https://doi.org/10.1016/j.cosust.2013.03.002, 2013.
Pappas, N., Roux, S., Hölzer, M., Lamkiewicz, K., Mock, F., Marz, M., and Dutilh B.E.: Virus Bioinformatics, Ref. Module Life Sci., 1, 124–132, https://doi.org/10.1016/B978-0-12-814515-9.00034-5, 2020.
Pesant, S., Not, F., Picheral, M., Kandels-Lewis, S., Le Bescot, N., Gorsky, G., Iudicone, D., Karsenti, E., Speich, S., Troublé, R., Dimier, C., and Searson, S.: Open science resources for the discovery and analysis of Tara Oceans data, Sci. Data, 2, 150023, https://doi.org/10.1038/sdata.2015.23, 2015.
Pörtner, H. O., Scholes, R. J., Arneth, A., Barnes, D. K. A., Burrows, M. T., Diamond, S. E., Duarte, C. M., Kiessling, W., Leadley, P., Managi, S., McElwee, P., Midgley, G., Ngo, H. T., Obura, D., Pascual, U., Sankaran, M., Shin, Y. J., and Val, A. L.: Overcoming the coupled climate and biodiversity crises and their societal impacts, Science, 380, eabl4881, https://doi.org/10.1126/science.abl4881, 2023.
Rantanen, M., Karpechko, A. Y., Lipponen, A., Nordling, K., Hyvarinen, O., Ruosteenoja, K., Vihma, T., and Laaksonen, A.: The Arctic has warmed nearly four times faster than the globe since 1979, Comm. Earth Environ., 3, 168, https://doi.org/10.1038/s43247-022-00498-3, 2022.
Roemmich, D. and the ARGO Steering Team: ARGO: the challenge of continuing 10 years of progress, Oceanogr., 22, 46–55, https://doi.org/10.2307/24860989, 2009.
Ropert-Coudert, Y., Kato, A., Wilson, R. P., and Cannell, B.: Foraging strategies and prey encounter rate of free-ranging Little Penguins, Mar. Biol., 149, 139–148, https://doi.org/10.1007/s00227-005-0188-x, 2006.
Royo-Llonch, M., Sánchez, P., Ruiz-González, C., Salazar, G., Pedrós-Alió, C., Sebastián, M., Labadie, K., Paoli, L. M., Ibarbalz, F., Zinger, L., Churcheward, B., Tara Oceans Coordinators, Chaffron, S., Eveillard, D., Karsenti, E., Sunagawa, S., Wincker, P., Karp-Boss, L., Bowler, C., and Acinas, S. G.: Compendium of 530 metagenome-assembled bacterial and archaeal genomes from the polar Arctic Ocean, Nat. Microbio., 6, 1561–1574, https://doi.org/10.1038/s41564-021-00979-9, 2021.
Sakamoto, K. Q., Sato, K., Ishizuka, M., Watanuki, Y., Takahashi, A., Daunt, F., and Wanless, S.: Can ethograms be automatically generated using body acceleration data from free-ranging birds?, PLoS ONE, 4, e5379, https://doi.org/10.1371/journal.pone.0005379, 2009.
Salzberg, S. L.: Next-generation genome annotation: we still struggle to get it right. Genome Biol., 20, 92, https://doi.org/10.1186/s13059-019-1715-2, 2019.
Schneider, M. V. and Orchard, S.: Omics Technologies, Data and Bioinformatics Principles, in: Bioinformatics for Omics Data, Methods in Molecular Biology, edited by: Mayer, B., Springer, Berlin, Germany, 3–30, https://doi.org/10.1007/978-1-61779-027-0_1, 2011.
Siegel, V.: Biology and ecology of Antarctic krill, Springer, Cham, Switzerland, ISBN 978-3-319-29277-9, 2016.
Smith, B., Fricker, H.A., Gardner, A.S., Medley, B., Nilsson, J., Paolo, F.S., Holschuh, N., Adusumilli, S., Brunt, K., and Zwally, H. J.: Pervasive ice sheet mass loss reflects competing ocean and atmosphere processes, Science, 368, 1239–1242, https://doi.org/10.1126/science.aaz5845, 2020.
Snijders, C., Matzat, U., and Reips, U.-D.: “Big Data”: Big Gaps of Knowledge in the Field of Internet Science. Inter. J. Internet Sci., 7, 1–5, 2012.
Steen, A. D., Kusch, S., Abdulla, H. A., Cakić, N., Coffinet, S., Dittmar, T., Fulton, J. M., Galy, V., Hinrichs, K.-U., Ingalls, A. E., Koch, B. P., Kujawinski, E., Liu, Z., Osterholz, H., Rush, D., Seidel, M., Sepúlveda, J., and Wakeham, S. G.: Analytical and Computational Advances, Opportunities, and Challenges in Marine Organic Biogeochemistry in an Era of “Omics”, Front. Mar. Sci., 7, 718, https://doi.org/10.3389/fmars.2020.00718, 2020.
Stow, C. A., Webster, K. E., Wagner, T., Lottig, N., Soranno, P. A., and Cha, Y. K.: Small values in big data: The continuing need for appropriate metadata, Ecol. Inform., 45, 26–30, https://doi.org/10.1016/j.ecoinf.2018.03.002, 2018.
Sunagawa, S., Acinas, S. G., Bork, P., Bowler, C., Tara Oceans Coordinators, Eveillard, D., Gorsky, G., Guidi, L., Iudicone, D., Karsenti, E., Lombard, F., Ogata, H., Pesant, S., Sullivan, M. B., Wincker, P., and de Vargas, C.: Tara Oceans: towards global ocean ecosystems biology, Nat. Rev. Microb., 18, 428–445, https://doi.org/10.1038/s41579-020-0364-5, 2020.
Sutton, G. J., Bost, C. A., Kouzani, A. Z., Adams, S. D., Mitchell, K., and Arnould, J. P.: Fine-scale foraging effort and efficiency of Macaroni penguins is influenced by prey type, patch density and temporal dynamics, Mar. Biol., 168, 1–16, https://doi.org/10.1007/s00227-020-03811-w, 2021.
Tanhua, T., Pouliquen, S., Hausman, J., O`Brien, K., Bricher, P., de Bruin, T., Buck, J. H., Burger, E. F., Carval, T., Casey, K. S., Diggs, S., Giorgetti, A., Glaves, H., Harscoat, V., Kinkade, D., Muelbert, J. H., Novellino, A., Pfeil, B., Pulsifer, P. L., Van de Putte, A., Robinson, E., Schaap, D., Smirnov, A., Smith, N., Snowden, D., Spears, T., Stall, S., Tacoma, M., Thijsse, P., Tronstad, S., Vandenberghe, T., Wengren, M., Wyborn, L., and Zhao, Z.: Ocean FAIR Data Services, Front. Mar. Sci., 6, 440, https://doi.org/10.3389/fmars.2019.00440, 2019.
Valletta, J., Torney, C., Kings, M., Thornton, A., and Madden, J.: Applications of machine learning in animal behaviour studies, Animal Behav., 124, 203–220, https://doi.org/10.1016/j.anbehav.2016.12.005, 2017.
Vernette, C., Lecubin, J., Sanchez, P., Tara Oceans Coordinators, Sunagawa, S., Delmont, T. O., Acinas, S. G., Pelletier, E., Hingamp, P., and Lescot, M.: The Ocean Gene Atlas v2.0: online exploration of the biogeography and phylogeny of plankton genes, Nucleic Acids Res., 50, 516–526, https://doi.org/10.1093/nar/gkac420, 2022.
Verwega, M. T., Trahms, C., Antia, A. N., Dickhaus, T., Prigge, E., Prinzler, M. H. U., Renz, M., Schartau, M., Slawig, T., Somes, C. J., and Biastoch, A.: Perspectives on Marine Data Science as a Blueprint for Emerging Data Science Disciplines, Front. Mar. Sci., 8, https://doi.org/10.3389/fmars.2021.678404, 2021.
Wille, J. D., Favier, V., Jourdain, N. C., Kittel, C., Turton, J. V., Agosta, C., Gorodetskaya, I. V., Picard, G., Codron, F., Leroy-Dos Santos, C., Amory, C., Fettweis, X., Blanchet, J., Jomelli, V., and Berchet, A.: Intense atmospheric rivers can weaken ice shelf stability at the Antarctic Peninsula, Comm. Earth Environ., 3, 90, https://doi.org/10.1038/s43247-022-00422-9, 2022.
Yandell, M. and Ence, D.: A beginner's guide to eukaryotic genome annotation, Nat. Rev. Genet., 13, 329–342, https://doi.org/10.1038/nrg3174, 2012.
Yang, C. and Huang, Q.: Spatial Cloud Computing, a Practical Approach, CRC Press, 357 pp., ISBN 9781138075559, 2013.
- Abstract
- General introduction
- State of the art
- Gaps and potential future gaps or challenges in Antarctic big data
- Solutions to fill the gaps in Antarctic big data
- Conclusions
- Data availability
- Author contributions
- Competing interests
- Disclaimer
- Acknowledgements
- Financial support
- Review statement
- References
Antarctic big data). For these large data sets there is a pressing need for improved data acquisition, curation, integration, service, and application to support fundamental scientific research, and this article describes and evaluates the current status of big data in various Antarctic scientific disciplines, identifies current gaps, and provides solutions to fill these gaps.
- Abstract
- General introduction
- State of the art
- Gaps and potential future gaps or challenges in Antarctic big data
- Solutions to fill the gaps in Antarctic big data
- Conclusions
- Data availability
- Author contributions
- Competing interests
- Disclaimer
- Acknowledgements
- Financial support
- Review statement
- References